ChatGPT, Large Language Models: the regulatory challenges of foundation models
28 June 2023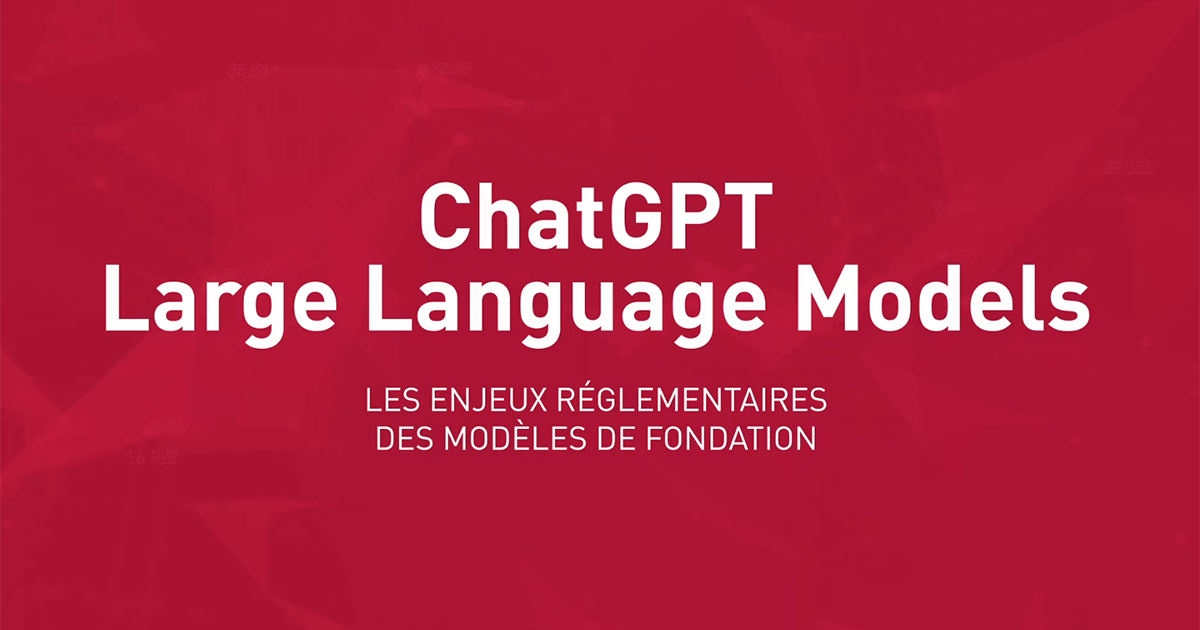
Winston Maxwell, Director of Law and Digital Studies at Télécom Paris, Institut Polytechnique de Paris, opened the webinar by introducing our speakers: Jules Pondard, AI expert at the ACPR, France; Karine Perset, director of the AI unit at the OECD; Félicien Vallet, head of the Technological Expertise Department at the CNIL, France; Léo Laugier, post-doctoral fellow at the École Polytechnique Fédérale de Lausanne, Switzerland. Our four experts shed light on the technical operation of foundation models, the political regulatory responses emerging around the world, data protection issues and the usefulness of LLMs (Large Language Models) for improving our online interactions. Below is a summary of their presentations.
As soon as it was released at the end of November 2022, ChatGPT, the conversational agent developed by Open AI, created a huge buzz among the general public. Other companies and researchers took the opportunity to unveil their own language models, such as Google’s « Bard », or Meta’s « Llama ». « Generative AI », which encompasses these text generation models as well as image and other content generators, has prompted a wave of reactions from major tech companies. For example, Google has initiated a « Code Red » and Microsoft has invested heavily in the Open AI company and called for the creation of an AI regulatory authority.
GPT-4 is already showing very impressive results, for example in American bar exams and medical school tests. Among its strengths are its writing, synthesis and computer programming skills. Its weaknesses include its dubious relationship with the truth as it sometimes seems to “hallucinate”, or its lack of ability to plan actions.
ChatGPT is built around three main blocks: (1) a neural network architecture based on the notion of « Transformers », which is called « Generative Pre-trained transformers (GPT) » in the case of ChatGPT, (2) a « Supervised Fined Tuning » stage, during which manually generated examples of questions and answers are used to modify the model, (3) a « Reinforcement Learning via Human Feedback » (RLHF) step, during which humans choose the most appropriate answer from several given by the model, for many different examples, thus teaching the model to give useful and harmless answers.
Many technical questions remain unanswered: What does the RLHF offer? How and why do these models work so well? Do they work well enough for real-life applications? How can they be audited and evaluated? We know that these models have limitations (lack of real understanding, etc.), but are they intrinsic to this type of model? Other debates concern the sovereignty of large companies versus open source, the protection of the data used for training, the biases present in this training data which is essentially American or English-speaking, and many other subjects.
Find out more
In May 2019, the OECD proposed ten principles for AI, which represent a set of priorities on AI policies and regulations, as well as the first international standard on AI. In June 2020, the G20 committed to using these principles, significantly expanding their geographical scope. The first 5 principles are values that AI systems should reflect, for example protecting human rights, equity and democratic values, or establishing stakeholder accountability. The next 5 principles are recommendations for fostering an AI ecosystem that can thrive and benefit societies in R&D and education policies, for example.
Since late November 2022, generative AI has been everywhere in the discussions of high-level political decision-makers. On May 20, G7 leaders established the « Hiroshima » process for generative AI, outlining their priorities on this topic. In particular, the G7 declaration emphasizes some of the OECD’s principles for AI. For example, it underlines the need to advance thinking and research on issues such as misinformation, lack of explainability, model safety and intellectual property issues (at a time when many lawsuits are starting in the USA on the latter subject). In addition, a wide range of national regulatory approaches are being developed, from soft law (US, EU, Japan and UK), to standards and risk frameworks such as the NIST framework in the US, to the use of existing regulations and regulators (US, UK, EU), and finally AI-specific regulation (EU, Canada).
The OECD has set up several initiatives on generative AI, such as a tracker of trends in generative AI-related investments, R&D and jobs and training (oecd.ai/trends-and-data); a catalog of AI risk assessment and AI governance tools (oecd.ai/tools); the creation of an anticipatory governance working group; regulatory experiments (sandboxes), particularly in the fields of privacy and finance; and real-time AI incident monitoring to prevent AI-related damage from recurring.
Find out more
- Generative AI and language models: opportunities, challenges and policy implications
- oecd.ai/classification: Gives a framework and a set of simple metrics for determining the key properties of an AI system concerning the data it uses, the model itself, its outputs, and its economic context.
On March 31, 2023, la Garante (the Italian data protection Authority) passed an emergency measure to ban ChatGPT in Italy. This decision was based on several factors, including the lack of information given to users whose data was used to train the model, the inaccuracy of certain data, and the absence of age verification. Having met some of the Garante’s requirements, ChatGPT was reinstated in Italy at the end of April. However a number of questions remain.
Firstly, what is the legal framework for processing personal data during the training of the model (we’re talking about training datasets of ~570Gb of text of varying quality for ChatGPT-4)? Secondly, is it possible to retrieve the personal data used for training from the model itself, for example via the mechanisms of inversion (an approximate version of a training data item is recreated), inference (a person’s association with a data group is inferred) or memorization (a personal data item memorized by the model is brought out). Finally, from the user interface, how can we exercise our right of access, rectification or deletion of transmitted information, or understand how data is processed?
Félicien Vallet proposes an analogy with the emergence of the right to oblivion in the context of search engines in the 2000s. This right to oblivion consists in deleting content from the results of a search engine, even if that content is still present on the web. A similar logic could be employed with LLMs: remove the content of a model’s results through filtering mechanisms without having to re-train the model.
To date, the CNIL has received 5 complaints concerning the protection of personal data on ChatGPT. These complaints are currently being processed. The CNIL has also published a dossier and an action plan on generative AI, aiming at framing the French and European development of generative AI in a way that respects privacy. At the European level, the EDPB Task Force on ChatGPT and LLMs is making progress on these issues.
Find out more
Automatic language processing (NLP) has undergone a paradigm shift over the past decade. For a long time, it was difficult for machines to understand the ambiguous nature (syntactic, contextual, lexical, etc.) of human language. But since around 2012, techniques such as Word2Vec have enabled language models to understand words in context and thus develop knowledge of language and of the world. This is known as the dispositional hypothesis: « you recognize a word by its companions » (Firth, 1957).
Léo Laugier’s work focuses on using language models to improve our online interactions.
A first example is the moderation of online messages. In this field, one of the difficulties is to detect certain insulting, impolite or « toxic » words in very long posts, for which the toxicity score might be slightly below the threshold beyond which the posts are deleted or analyzed. This task is known as Toxic Span detection. Leo’s work focused on the use of weakly supervised learning to solve this task.
Another example is the reformulation of offensive language, with the aim of encouraging calmer, more constructive conversations. The idea behind the method is that of translation, where the machine learns to transfer from one language to another, in this case from toxic to civic language. However, there are risks of hallucination (the model produces false content), supererogation (the model adds something) or position reversal (the model gives an opposite meaning to the initial sentence).
Léo is currently studying online polarization at the EPFL, with the aim of protecting democracy from the threat of online radicalization and polarization, without undermining pluralism.
- Les modèles de fondation : des solutions pour améliorer nos interactions en ligne ? (French)
- perspectiveapi.com
- SemEval-2021 task 5: Toxic spans detection Pavlopoulos et al., 2021
- Civil rephrases of toxic texts with self-supervised transformers Laugier et al., 2021
- https://www.kaggle.com/code/arunmohan003/question-answering-using-bert
In conclusion, Olivier Fliche, Director of the ACPR’s Fintech Innovation Unit, highlighted some of the critical issues introduced in this webinar. Some are not new, such as transparency, explainability and governance. Others have emerged with the arrival of generative AI, such as the question of the accuracy of generated content. In addition, research into human-computer interaction highlights the tendency to anthropomorphize these conversational tools, which can lead to overconfidence in their predictions.
View the replay (in French)
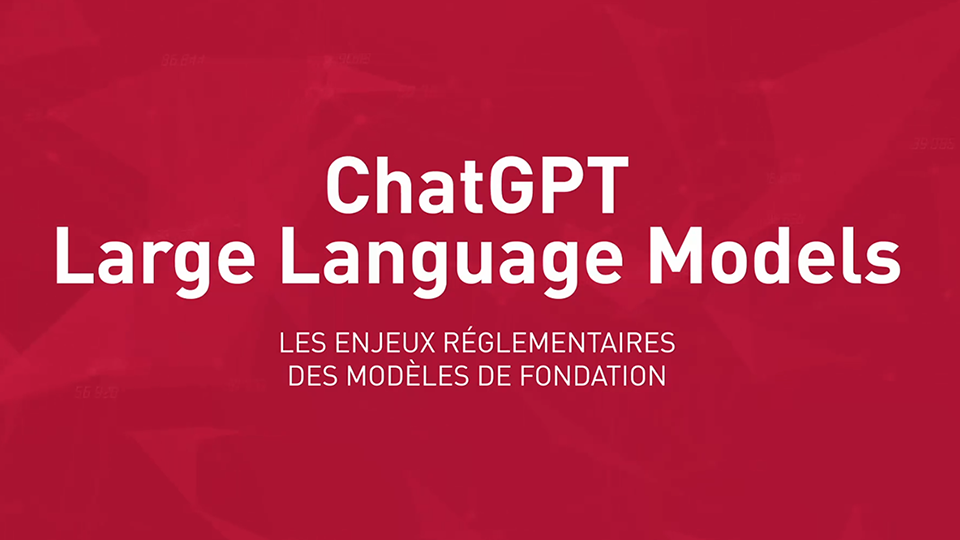